Beyond the Algorithm Understanding AI Bias
Explore the causes and consequences of AI bias, and learn about strategies to mitigate its effects on fairness and equity in AI systems.
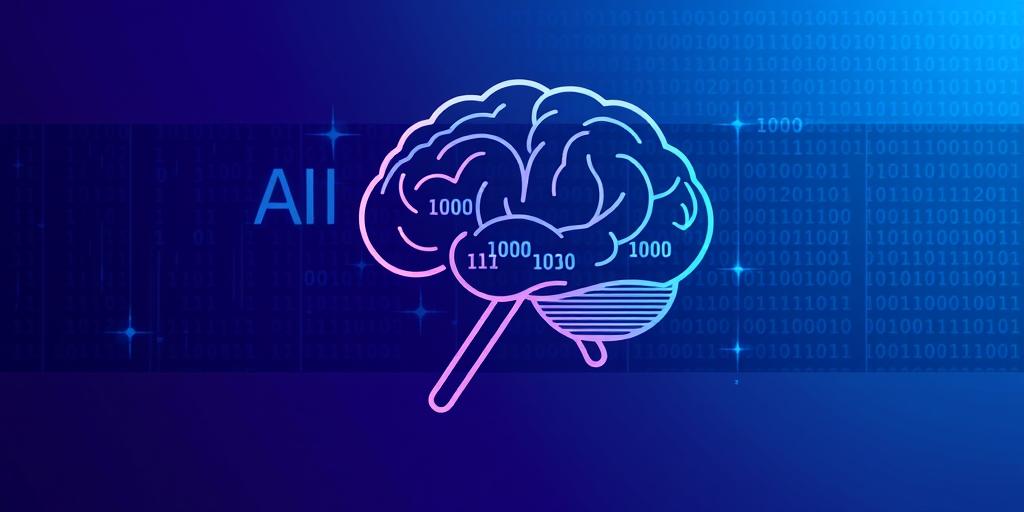
Beyond the Algorithm Understanding AI Bias
AI bias is a pervasive issue that can have significant consequences across various applications. It arises when algorithms are trained on data that reflects existing societal biases, leading to skewed or discriminatory outcomes. Understanding the sources of AI bias and implementing strategies to mitigate it are crucial for ensuring fairness and equity in AI systems.
One of the primary sources of AI bias is biased training data. If the data used to train an AI model does not accurately represent the population it will be used to make decisions about, the model is likely to produce biased results. For example, if a facial recognition system is trained primarily on images of one race, it may perform poorly when identifying individuals of other races. Another source of AI bias is flawed algorithm design. If the algorithm is designed in a way that systematically favors certain groups over others, it will produce biased results, even if the training data is unbiased. Finally, AI bias can also result from biased human input. If humans are involved in the process of labeling or curating data, their own biases can creep into the data and influence the model's output.
Several strategies can be employed to mitigate AI bias. One strategy is to use more diverse and representative training data. This involves collecting data from a wide range of sources and ensuring that all relevant groups are adequately represented. Another strategy is to use bias detection and mitigation techniques. These techniques can help identify and correct biases in both the training data and the algorithm itself. Finally, it is important to promote transparency and accountability in AI systems. This means making the decision-making process of AI systems more transparent and holding developers accountable for the biases that their systems produce. By understanding the sources of AI bias and implementing strategies to mitigate it, we can ensure that AI systems are fair, equitable, and beneficial for all.